Genomics
Dissecting the biological bases of disease
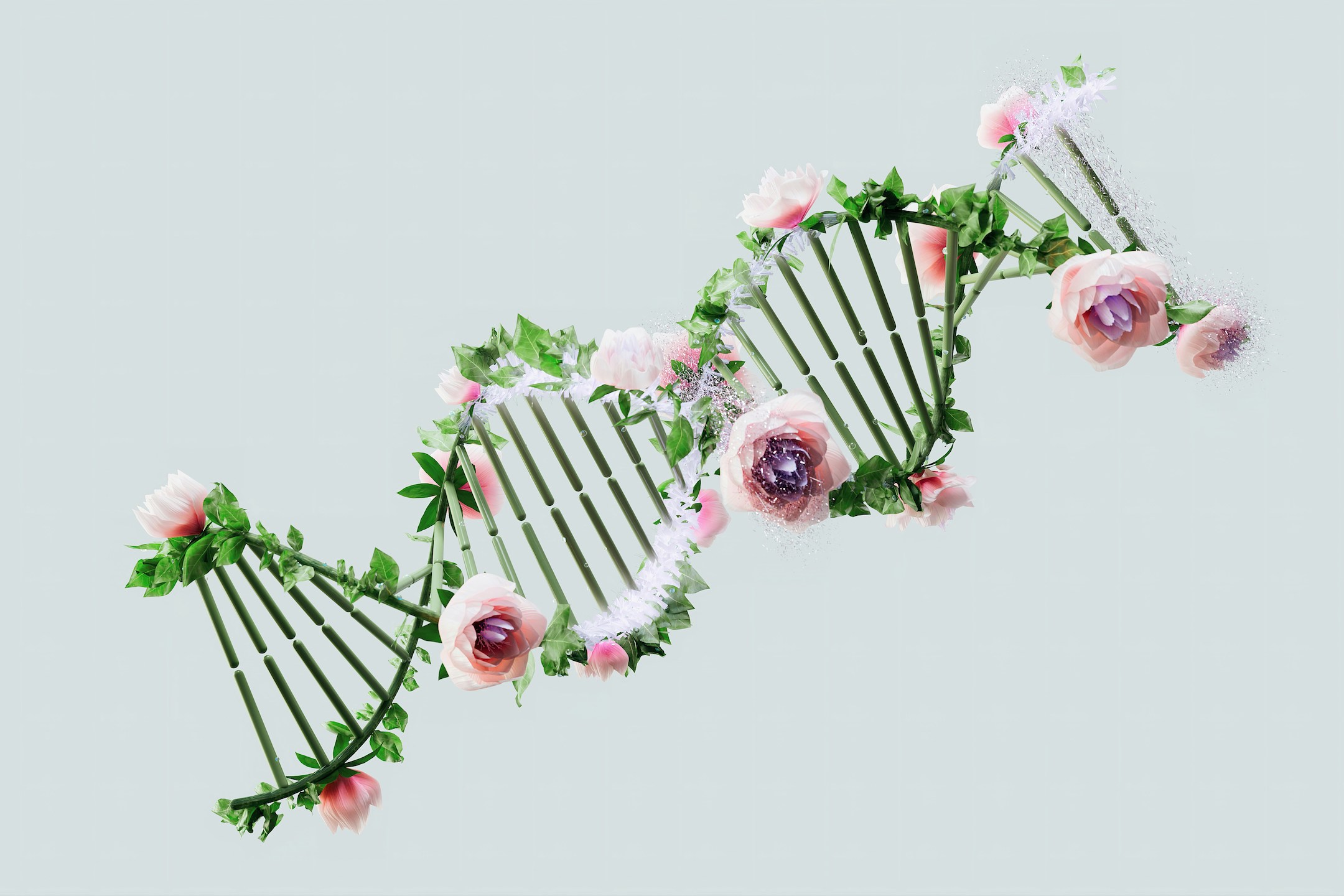
Genomics and epigenetics provide high-resolution views of the diversity and heterogeneity of populations at the cellular level. We are building computational tools to transform these observations into understanding of disease mecahnisms.
References
2023
- Integrating single-cell RNA-seq datasets with substantial batch effectsKarin Hrovatin, Amir Ali Moinfar, Alejandro Tejada Lapuerta, and 4 more authors26–28 aug 2023
@article{hrovatin2023evaluation, author = {Hrovatin, Karin and Ali Moinfar, Amir and Lapuerta, Alejandro Tejada and Zappia, Luke and Lengerich, Ben and Kellis, Manolis and Theis, Fabian J.}, title = {Integrating single-cell RNA-seq datasets with substantial batch effects}, year = {2023}, }
2022
- Ten quick tips for deep learning in biologyBenjamin D Lee, Anthony Gitter, Casey S Greene, and 17 more authorsPLoS computational biology, 26–28 aug 2022
@article{lee2022ten, title = {Ten quick tips for deep learning in biology}, author = {Lee, Benjamin D and Gitter, Anthony and Greene, Casey S and Raschka, Sebastian and Maguire, Finlay and Titus, Alexander J and Kessler, Michael D and Lee, Alexandra J and Chevrette, Marc G and Stewart, Paul Allen and Britto-Borges, Thiago and Cofer, Evan M. Cofer and Yu, Kun-Hsing and Carmona, Juan Jose and Fertig, Elana J. and Kalinin, Alexandr A. and Signal, Brandon and Lengerich, Benjamin J. and Triche, Timothy J. Jr. and Boca, Simina M.}, journal = {PLoS computational biology}, informal_venue = {PLoS CompBio}, volume = {18}, number = {3}, pages = {e1009803}, year = {2022}, publisher = {Public Library of Science San Francisco, CA USA}, keywords = {Deep Learning, Biology, Computational Genomics}, }
2018
- Precision Lasso: Accounting for Correlations and Linear Dependencies in High-Dimensional Genomic DataBioinformatics, 26–28 aug 2018
Association studies to discover links between genetic markers and phenotypes are central to bioinformatics. Methods of regularized regression, such as variants of the Lasso, are popular for this task. Despite the good predictive performance of these methods in the average case, they suffer from unstable selections of correlated variables and inconsistent selections of linearly dependent variables. Unfortunately, as we demonstrate empirically, such problematic situations of correlated and linearly dependent variables often exist in genomic datasets and lead to under-performance of classical methods of variable selection. To address these challenges, we propose the Precision Lasso. Precision Lasso is a Lasso variant that promotes sparse variable selection by regularization governed by the covariance and inverse covariance matrices of explanatory variables. We illustrate its capacity for stable and consistent variable selection in simulated data with highly correlated and linearly dependent variables. We then demonstrate the effectiveness of the Precision Lasso to select meaningful variables from transcriptomic profiles of breast cancer patients. Our results indicate that in settings with correlated and linearly dependent variables, the Precision Lasso outperforms popular methods of variable selection such as the Lasso, the Elastic Net and Minimax Concave Penalty (MCP) regression.
@article{wang2018precision, title = {Precision Lasso: Accounting for Correlations and Linear Dependencies in High-Dimensional Genomic Data}, author = {Wang, Haohan and Lengerich, Benjamin J. and Aragam, Bryon and Xing, Eric P}, journal = {Bioinformatics}, volume = {35}, number = {7}, pages = {1181--1187}, year = {2018}, informal_venue = {Bioinformatics}, publisher = {Oxford University Press}, keywords = {Statistical Genetics, Genomics}, }
- Personalized Regression Enables Sample-specific Pan-cancer AnalysisBioinformatics, 26–28 aug 2018
In many applications, inter-sample heterogeneity is crucial to understanding the complex biological processes under study. For example, in genomic analysis of cancers, each patient in a cohort may have a different driver mutation, making it difficult or impossible to identify causal mutations from an averaged view of the entire cohort. Unfortunately, many traditional methods for genomic analysis seek to estimate a single model which is shared by all samples in a population, ignoring this inter-sample heterogeneity entirely. In order to better understand patient heterogeneity, it is necessary to develop practical, personalized statistical models. To uncover this inter-sample heterogeneity, we propose a novel regularizer for achieving patient-specific personalized estimation. This regularizer operates by learning two latent distance metrics—one between personalized parameters and one between clinical covariates—and attempting to match the induced distances as closely as possible. Crucially, we do not assume these distance metrics are already known. Instead, we allow the data to dictate the structure of these latent distance metrics. Finally, we apply our method to learn patient-specific, interpretable models for a pan-cancer gene expression dataset containing samples from more than 30 distinct cancer types and find strong evidence of personalization effects between cancer types as well as between individuals. Our analysis uncovers sample-specific aberrations that are overlooked by population-level methods, suggesting a promising new path for precision analysis of complex diseases such as cancer.
@article{lengerich2018personalized, author = {Lengerich, Benjamin J. and Aragam, Bryon and Xing, Eric P}, title = {Personalized Regression Enables Sample-specific Pan-cancer Analysis}, journal = {Bioinformatics}, volume = {34}, number = {13}, pages = {i178-i186}, year = {2018}, informal_venue = {ISMB}, doi = {10.1093/bioinformatics/bty250}, url = {http://dx.doi.org/10.1093/bioinformatics/bty250}, eprint = {/oup/backfile/content_public/journal/bioinformatics/34/13/10.1093_bioinformatics_bty250/1/bty250.pdf}, keywords = {Interpretable, Contextualized, Statistical Genetics, Genomics, Cancer}, }
- Opportunities and Obstacles for Deep Learning in Biology and MedicineTravers Ching, Daniel S. Himmelstein, Brett K. Beaulieu-Jones, and 33 more authorsJournal of The Royal Society Interface, 26–28 aug 2018
Deep learning describes a class of machine learning algorithms that are capable of combining raw inputs into layers of intermediate features. These algorithms have recently shown impressive results across a variety of domains. Biology and medicine are data-rich disciplines, but the data are complex and often ill-understood. Hence, deep learning techniques may be particularly well suited to solve problems of these fields. We examine applications of deep learning to a variety of biomedical problems—patient classification, fundamental biological processes and treatment of patients—and discuss whether deep learning will be able to transform these tasks or if the biomedical sphere poses unique challenges. Following from an extensive literature review, we find that deep learning has yet to revolutionize biomedicine or definitively resolve any of the most pressing challenges in the field, but promising advances have been made on the prior state of the art. Even though improvements over previous baselines have been modest in general, the recent progress indicates that deep learning methods will provide valuable means for speeding up or aiding human investigation. Though progress has been made linking a specific neural network’s prediction to input features, understanding how users should interpret these models to make testable hypotheses about the system under study remains an open challenge. Furthermore, the limited amount of labelled data for training presents problems in some domains, as do legal and privacy constraints on work with sensitive health records. Nonetheless, we foresee deep learning enabling changes at both bench and bedside with the potential to transform several areas of biology and medicine.
@article{ching2018opportunities, author = {Ching, Travers and Himmelstein, Daniel S. and Beaulieu-Jones, Brett K. and Kalinin, Alexandr A. and Do, Brian T. and Way, Gregory P. and Ferrero, Enrico and Agapow, Paul-Michael and Zietz, Michael and Hoffman, Michael M. and Xie, Wei and Rosen, Gail L. and Lengerich, Benjamin J. and Israeli, Johnny and Lanchantin, Jack and Woloszynek, Stephen and Carpenter, Anne E. and Shrikumar, Avanti and Xu, Jinbo and Cofer, Evan M. and Lavender, Christopher A. and Turaga, Srinivas C. and Alexandari, Amr M. and Lu, Zhiyong and Harris, David J. and DeCaprio, Dave and Qi, Yanjun and Kundaje, Anshul and Peng, Yifan and Wiley, Laura K. and Segler, Marwin H. S. and Boca, Simina M. and Swamidass, S. Joshua and Huang, Austin and Gitter, Anthony and Greene, Casey S.}, title = {Opportunities and Obstacles for Deep Learning in Biology and Medicine}, volume = {15}, number = {141}, year = {2018}, doi = {10.1098/rsif.2017.0387}, publisher = {The Royal Society}, informal_venue = {JRSI}, issn = {1742-5689}, url = {http://rsif.royalsocietypublishing.org/content/15/141/20170387}, eprint = {http://rsif.royalsocietypublishing.org/content/15/141/20170387.full.pdf}, journal = {Journal of The Royal Society Interface}, keywords = {Deep Learning, Biology, Computational Genomics}, }